Writing a dissertation or capstone project is no easy feat. It requires countless hours of research, data collection, and analysis. One of the most critical aspects of any dissertation is the data analysis phase. This is where you delve deep into your collected data to uncover meaningful insights and draw well-supported conclusions. However, mastering dissertation data analysis can be a daunting task for many students.
Data analysis involves more than just running statistical tests or creating charts and graphs. It requires a comprehensive understanding of the research questions, study design, and the specific data set being analyzed. Whether you are working with quantitative or qualitative data, the goal is to make sense of the information you have gathered and transform it into meaningful findings.
In the world of dissertations and capstones, data analysis is like cracking a code. It involves deciphering the patterns and relationships within your data, uncovering hidden insights, and constructing a narrative that adds value to your research. The process can be both challenging and rewarding, as it allows you to bring your research to life and contribute to the existing body of knowledge in your field.
In this article, we will guide you through the intricate process of dissertation data analysis. We will explore various techniques, tools, and approaches that will help you master this crucial aspect of your research. Whether you are a novice researcher or an experienced scholar, we hope to provide you with practical tips and insights that will enhance your data analysis skills. So, let’s dive in and unlock the secrets of effective dissertation data analysis!
Understanding Dissertation Data Analysis
In the world of academic research, dissertations and capstones play a crucial role in the advancement of knowledge. These scholarly works require rigorous analysis to derive meaningful insights and draw valid conclusions. This is where dissertation data analysis comes into the picture. It is a systematic process of examining and interpreting the data collected during research, providing a solid foundation for the findings presented in a dissertation.
Dissertation data analysis involves transforming raw data into a format that is more manageable and conducive to analysis. This can include organizing data sets, cleaning and filtering out irrelevant information, and preparing the data for statistical or qualitative analysis methods. By applying appropriate techniques, researchers gain the ability to extract meaningful patterns, trends, and relationships from the data, enhancing the validity and reliability of their findings.
One of the key objectives of dissertation data analysis is to uncover significant findings that contribute to the existing knowledge base. Through careful examination and interpretation of the collected data, researchers can identify patterns, make comparisons, and draw inferences that shed light on the research problem being addressed. This process helps to validate the research hypotheses or research questions and provides insightful answers to the research problem. Dissertation data analysis, therefore, serves as the backbone of a well-structured and evidence-based academic work.
MBA dissertation help
In conclusion, understanding and mastering dissertation data analysis is crucial for anyone embarking on a research journey. It forms the basis for drawing meaningful insights, validating research hypotheses, and contributing to the body of knowledge in various academic disciplines. By following a systematic approach to analyzing data, researchers can ensure the reliability and validity of their research findings, ultimately making a significant impact in their respective fields.
Key Methods for Data Analysis in Dissertations
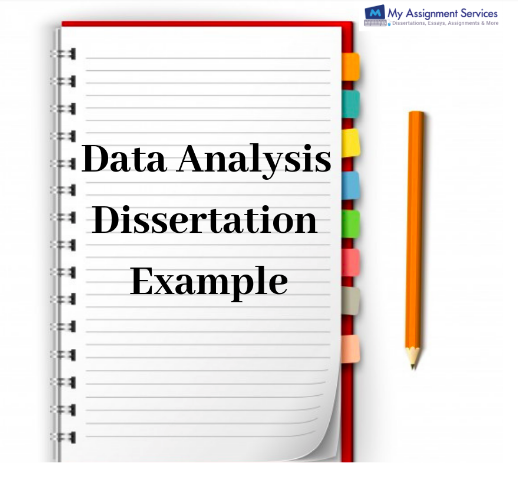
In dissertations and capstones, data analysis plays a crucial role in uncovering meaningful insights and drawing valid conclusions. This section will explore some of the key methods used for data analysis in the context of dissertations, focusing on their relevance and potential applications.
1. Quantitative Analysis: For dissertations that involve numerical data, quantitative analysis is often employed to examine patterns, relationships, and trends. This method involves applying statistical techniques to quantify and interpret data, enabling researchers to draw objective conclusions. Whether it’s conducting surveys, experiments, or analyzing large datasets, quantitative analysis provides a systematic approach to measuring variables and testing hypotheses.
2. Qualitative Analysis: Dissertations also frequently utilize qualitative analysis to explore in-depth insights and delve into complex phenomena. This method involves interpreting textual, visual, or audio data to uncover themes, patterns, and meanings. Researchers conduct interviews, focus groups, or analyze existing data sources to generate rich descriptions and gain a deeper understanding of the research topic. Qualitative analysis helps capture valuable perspectives, experiences, and narratives that quantitative methods alone may struggle to uncover.
3. Mixed-Methods Analysis: Some dissertations opt for a mixed-methods approach, combining both quantitative and qualitative analysis to capitalize on the strengths of each method. This approach provides a more comprehensive and robust perspective, allowing researchers to triangulate their findings and gain a more nuanced understanding of their research question. By integrating different types of data and analysis techniques, mixed-methods analysis can offer a more holistic view of the research topic, bridging the gap between numbers and narratives.
As each dissertation is unique, the choice of methods for data analysis depends on various factors such as research objectives, data type, and disciplinary norms. An effective data analysis strategy involves careful consideration of these factors to ensure the chosen methods align with the research aims and maximize the overall rigor and validity of the study.
Best Practices for Successful Dissertation Data Analysis
In order to excel in dissertation data analysis, there are several best practices you should keep in mind. By following these guidelines, you can ensure a smoother and more effective analysis process for your dissertations and capstones.
Firstly, it is crucial to begin by clearly outlining your research questions and objectives. This will help you stay focused throughout the data analysis process and ensure that you are extracting relevant insights. Take the time to carefully review your research goals and design your analysis plan accordingly.
Once you have a clear direction, it’s important to organize and clean your data before diving into analysis. Data cleansing involves identifying and rectifying any errors, inconsistencies, or missing values in your dataset. Ensuring data accuracy and integrity will lead to more reliable and valid results.
Next, when conducting your analysis, it is advisable to utilize a combination of quantitative and qualitative techniques. Quantitative analysis involves statistical methods, while qualitative analysis focuses on interpreting textual or visual data. By seamlessly integrating both approaches, you can gain a comprehensive understanding of your findings.
Lastly, effective communication of your results is key. It is essential to present your analysis in a clear and concise manner, making it accessible to both experts and non-experts in your field. Visualizations, tables, and charts can help convey your findings effectively, allowing for easy comprehension and interpretation.
By adhering to these best practices, you can master the art of dissertation data analysis and produce impactful research outcomes. Remember to stay organized, clean your data, employ diverse analysis techniques, and effectively communicate your results for a successful dissertation experience.